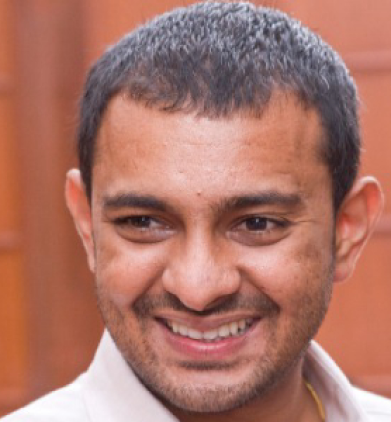
Dr. Nandan Sudarsanam
Professor

Ph.D.(Engineering Systems Division) ,Massachusetts Institute of Technology (MIT), Cambridge, MA, 2008

M.S.(Industrial Engineering & Management) , Oklahoma State University, Stillwater, OK, 2005

B.E (Mechanical Engineering), Shanmugha College of Engineering, Thanjavur, India, 2003

+91-44-22574580

nandan[at]iitm[.]ac[.]in

Office : DoMS 306
- Experimentation
- Machine learning/ Data Mining
- Applied Statistics
- Algorithmic and Heuristic approaches to problem solving
- Paramasivan, K., Raj, B., Sudarasanam, N., & Subburaj, R. (2023). Prolonged school closure during the pandemic time in successive waves of COVID-19- vulnerability of children to sexual abuses - A case study in Tamil Nadu, India. Heliyon, 9(7), e17865.
- Rammohan, S., Marathe, R.R. & Sudarsanam, N. Recent advancements in revenue management of taxi services: a systematic review and research agenda. Management Review Quaterly (2023). https://doi.org/10.1007/s11301-023-00326-8
- Paramasivan, K., Subramani, B., & Sudarsanam, N. (2022). Counterfactual analysis of the impact of the first two waves of the COVID-19 pandemic on the reporting and registration of missing people in India. Humanities and Social Sciences Communications, 9(1), 1-14.
- Rammohan, S., Marathe, R. R., & Sudarsanam, N. (2022). Profitable market mechanism for platform-based aggregator taxi services. Transportation Research Interdisciplinary Perspectives, 16, 100687.
- Sudarsanam, N., Kumar, A., & Frey, D. D. (2022). Quantifying the maximum possible improvement in 2^k experiments. Research in Engineering Design, 33(4), 367-384.
- Paramasivan, K., Subburaj, R., Jaiswal, S., & Sudarsanam, N. (2022). Empirical evidence of the impact of mobility on property crimes during the first two waves of the COVID-19 pandemic. Humanities and Social Sciences Communications, 9(1), 1-14.
- Narayanaswami, Sai Kiran, Nandan Sudarsanam, and Balaraman Ravindran. "An Active Learning Framework for Efficient Robust Policy Search." 5th Joint International Conference on Data Science & Management of Data (9th ACM IKDD CODS and 27th COMAD). 2022.
- Paramasivan, K., Subburaj, R., Sharma, V. M., & Sudarsanam, N. (2022). Relationship between mobility and road traffic injuries during COVID-19 pandemic—The role of attendant factors. PLoS one, 17(5).https://doi.org/10.1371/journal.pone.0268190
- Paramasivan, K., Sudarsanam, N., Vellaichamy, S., Norris, K. K., & Subburaj, R. (2022). Crime registration and distress calls during COVID-19: two sides of the coin. Policing and Society, 1–22. https://doi.org/10.1080/10439463.2021.2023526
- Paramasivan, K., & Sudarsanam, N. (2021). Impact of COVID-19 pandemic on road safety in Tamil Nadu, India. International Journal of Injury Control and Safety Promotion. https://doi.org/10.1080/17457300.2021.2007134
- Muralidharan, V., Sudarsanam, N., & Ravindran, B. (2021). Inferring customer occupancy status in for-hire vehicles using PU Learning. In 8th ACM IKDD CODS and 26th COMAD (pp. 290-298).
- Venkatraman, K., Vijayalakshmi, V., Sudarsanam, N., & Manoharan, A. (2020). "Designing Dynamic Interventions to Improve Adherence in Pediatric Long-term Treatment–The Role of Perceived Value of the Physician by Primary Caregivers". Health Communication, 1-16.
- Sudarsanam, N., Chandran, R., & Frey, D. D. (2020), "Conducting non-adaptive experiments in a live setting: a Bayesian approach to determining optimal sample size" ASME Journal of Mechanical Design, 142(3).
- Sudarsanam, Nandan, Nishanth Kumar, Abhishek Sharma, and Balaraman Ravindran, (2020),"Rate of change analysis for interestingness measures." Knowledge and Information Systems 62, no. 1: 239-258.
- Sudarsanam, Nandan, Balaji Pitchai Kannu, and Daniel D. Frey, (2019) "Optimal replicates for designed experiments under the online framework." Research in Engineering Design 30, no. 3 : 363-379.
- Mukherjee, S., Naveen, K. P., Sudarsanam, N., & Ravindran, B. (2018). Efficient-UCBV: An Almost Optimal Algorithm Using Variance Estimates. In Thirty-Second AAAI Conference on Artificial Intelligence.
- Philip, D. J., Sudarsanam, N., & Ravindran, B. (2018). Improved Insights on Financial Health through Partially Constrained Hidden Markov Model Clustering on Loan Repayment Data. ACM SIGMIS Database: the DATA BASE for Advances in Information Systems, 49(3), 98-113.
- Sudarsanam, Nandan, and Balaraman Ravindran, (2018) "Using Linear Stochastic Bandits to extend traditional offline Designed Experiments to online settings." Computers & Industrial Engineering 115: 471-485.
- Mukherjee, S., Naveen, K. P., Sudarsanam, N., and Ravindran, B. (2017) "Thresholding Bandits with Augmented UCB". In the Proceedings of the Twenty Sixth International Joint Conference on Artificial Intelligence (IJCAI 2017).
- Sudarsanam, N., and Frey D. D., (2011), “Using Ensemble Techniques to advance Adaptive-One-Factor-at-a-Time Experimentation”, Quality and Reliability Engineering International, Vol. 27, Is 7, pg 947-957.
- Frey, D. D., and Sudarsanam, N., (2006), “Adaptive One-factor-at-a-time Method for Robust Parameter Design: Comparison with Crossed Arrays via Case Studies”, ASME Journal of Mechanical Design, Vol. 130, Is. 2, pp. 02140-14.
- Li, X., Sudarsanam, N., and Frey, D. D., (2006), “Regularities in Data from factorial experiments”, Complexity, Vol.11, Is. 5, pp 32-45.
- Kumar, A. and Sudarsanam N., 2019, “Automated Kano Model Categorization of Aspects from Online Ratings”, International Conference on Computers and Industrial Engineering, (CIE-48), Auckland, New Zealand. December 2-5.
-
Sudarsanam, N., et al., 2017, "Optimal sample size for A/B tests using cumulative regret", Conference on Reinforcement Learning and Decision Making (RLDM), Ann Arbor, Michigan, June 11-14.
-
Sudarsanam N., and Philip D., 2016, "Quantifying and Predicting Prepayments in the Microfinance Environment", NSE-IFMR Finance Foundation Conference on Household Finance, Mumbai, India, March 14-15.
-
Sudarsanam, N., et al., 2015, "Bootstrapped Linear Bandits", Conference on Reinforcement Learning and Decision Making (RLDM), Alberta, Canada, June 7-10.
- Rackson Asset Management Llc, New York, NY – 2009-2013: Quantitative Research at a high frequency, algorithmic trading environment
- Bank of America, Boston, MA – Winter 2008
- Ford Motor Company, Detroit, MI – Summer 2006